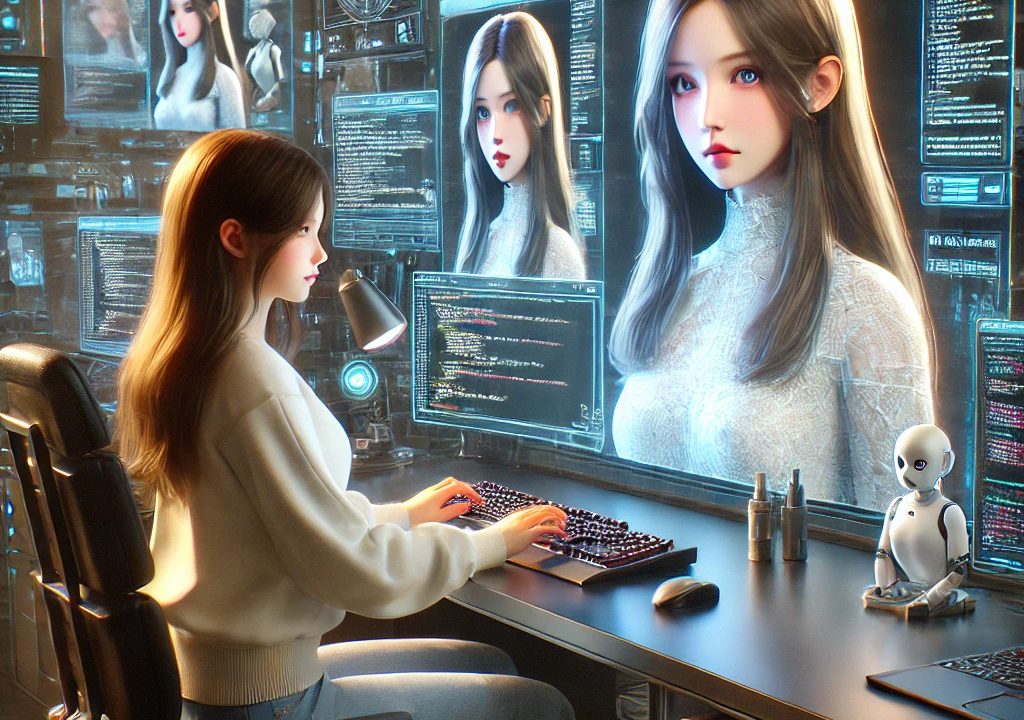
Revolutionizing AI: How META’s Self-Taught Evaluator is Shaping the Future of Autonomous Coding
Farhad Reyazat – PhD in Risk Management , Fintech & Ai University of Oxford ( Biography)
Artificial Intelligence (AI) has come a long way from its inception. With the rapid advancements in technology, we are witnessing machines that don’t just follow commands but learn, adapt, and improve independently. Enter META’s Self-Taught Evaluator, an innovation that has the potential to redefine how we think about AI, coding, and the future of machine learning. Imagine an AI capable of writing its code, evaluating its work, and improving autonomously—all without human intervention. This futuristic concept is no longer a dream; it’s happening now.
AI’s Evolution in Coding: From Assistant to Autonomous
Over the past decade, AI has been integrated into the healthcare and entertainment industries. It has helped doctors diagnose diseases, recommended products to online shoppers, and even suggested your next Netflix binge-watch. However, regarding coding, AI has mostly played a supporting role. Tools like GitHub Copilot have made waves by assisting developers with code completion, debugging, and optimization. These tools significantly reduce errors and save time, but they’ve always needed humans in the driver’s seat.
But what if we flipped the script? What if AI could take complete control, writing, testing, and improving its code? That’s precisely what META’s Self-Taught Evaluator does. This leap forward in AI development positions machines as helpers and autonomous coders capable of independent growth and optimization.
For example, a recent report from OpenAI stated that their code-generating model Codex (which powers GitHub Copilot) had been integrated into more than 3,000 apps and was handling up to 30% of code in languages like Python during its early testing phase. Yet, even Codex required continuous human input for refining and debugging. META’s Self-Taught Evaluator, however, aims to remove the need for constant human supervision.
How Does META’s Self-Taught Evaluator Work?
At its core, META’s Self-Taught Evaluator uses a seed language model—a pre-trained model that already understands human-preferred outputs. From here, the evaluator dives into a vast pool of human-written tasks, which could be anything from simple coding challenges to real-world software development problems. The AI generates two responses for each task—one good and one subpar—labeling them “chosen” and “rejected.” It then iterates through multiple rounds, refining its reasoning and learning from its mistakes. Once it perfects its reasoning, the successful examples are added to its training set, and the AI improves with each pass.
One of the most groundbreaking aspects of the Self-Taught Evaluator is that it learns similarly to humans—by analyzing mistakes and refining its approach. However, unlike humans, this AI doesn’t require rest, coffee, or breaks. It operates continuously, optimizing its capabilities at an exponential rate. Over time, it builds a robust dataset of what works and what doesn’t, allowing it to fine-tune itself based on real-world examples.
Real-World Success: META’s AI Goes Beyond Benchmarks
META’s team has already tested the Self-Taught Evaluator with impressive results. Using their LLAMA370B Instruct model—a robust machine-learning framework—they measured performance using the RewardBench Benchmark, a tool designed to assess how well AI models perform various tasks. After five rounds of self-teaching, the model’s accuracy jumped from 75.4% to a remarkable 88.7%.
To put this into perspective, this entire process was completed without human input. There were no expert annotations, no manual tweaking—just the AI learning, refining, and improving by itself.
But it doesn’t stop there. The Self-Taught Evaluator also excelled in the MTBench Benchmark, which evaluates the AI’s ability to handle multi-turn conversations—a crucial skill for chatbots, virtual assistants, and other applications where understanding context is critical. In some cases, the Self-Taught Evaluator even outperformed models that human experts had fine-tuned.
The implications for businesses are enormous. Faster deployment, reduced costs, and fewer development resources are all possible. Take the case of a chatbot system in customer service. Typically, such systems require extensive human oversight for initial training and ongoing refinement. With META’s technology, businesses can feed vast amounts of unlabeled data, and the AI can learn and improve independently. This drastically cuts down the time and expense usually associated with AI training.
Why Businesses Should Care
One of the most significant bottlenecks in AI deployment has always been the need for labeled data, which human experts usually annotate. This process takes time and money. In fact, according to a study by Cognilytica, up to 80% of AI project time is spent on data labeling and preparation, delaying the implementation of AI solutions by months. META’s Self-Taught Evaluator bypasses this hurdle by allowing businesses to use unlabeled data—customer interactions, product reviews, or even legal documents—enabling the AI to train itself. The result? Faster rollouts, lower costs, and more time for teams to focus on high-priority tasks.
Moreover, the Self-Taught Evaluator’s ability to self-improve means it can be used across various industries, from finance to healthcare to retail. It’s like having a super-smart intern who can analyze data, optimize supply chains, or predict customer sentiment without constant supervision. For instance, without human intervention, a retailer could use this AI to analyze vast amounts of customer data, identify patterns, and improve marketing strategies in real-time.
The Challenges and Future of Self-Taught AI
As exciting as this technology is, it’s essential to approach it with a balanced perspective. The success of the Self-Taught Evaluator hinges on the quality of the seed model you begin with. If the seed model is flawed or poorly aligned with the task, the AI might excel in tests but fail in real-world scenarios. Additionally, while the need for human oversight is reduced, it’s not eliminated. Businesses will still need to perform manual checks at various stages to ensure the AI remains on track and doesn’t veer into “overfitting” on benchmarks, which can make it less effective in practice.
Despite these challenges, META’s Self-Taught Evaluator represents a monumental leap forward in AI development. It opens the door to a future where AI assists and autonomously drives innovation. Imagine a world where AI systems adapt in real-time, learning from their environment and continuously improving, from more capable virtual assistants to AI systems that can predict needs and anticipate trends with unprecedented accuracy.
META’s innovation could push AI into entirely new territories as we move forward, blurring the line between human and machine problem-solving. The next generation of AI will be intelligent and self-sufficient, and that changes everything.
No Comments