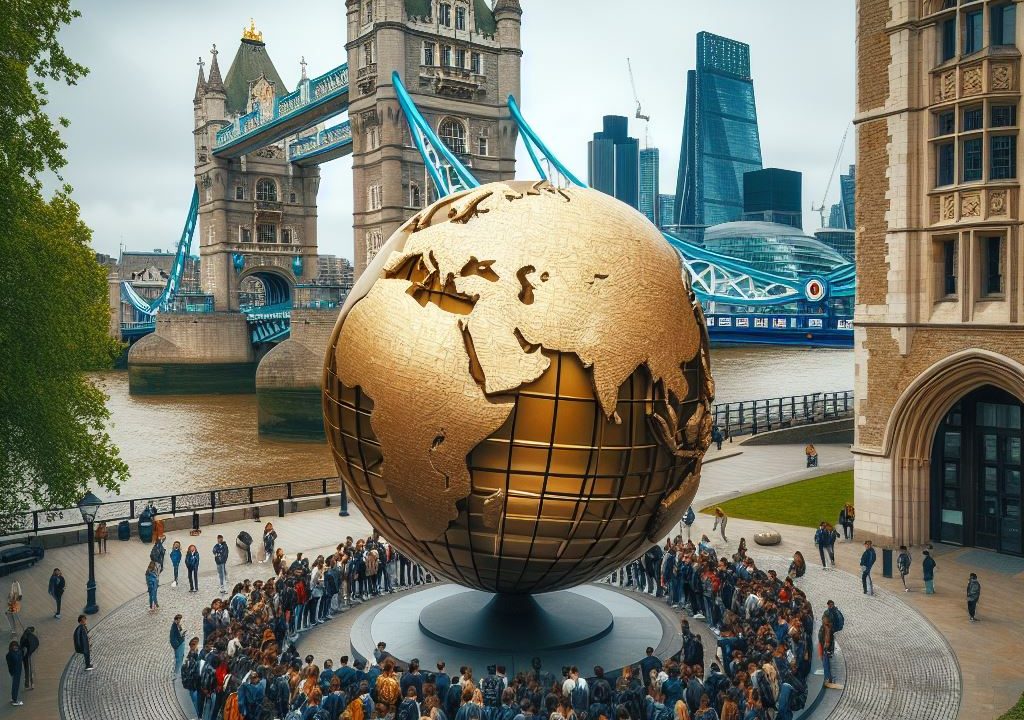
Quantum Leap AI: How Gen AI Optimizes Portfolio Structure and Investment Strategies
Dr Farhad Reyazat – London School of Banking & Finance
7th of March 2024
Citation:Reyazat, F. (2024, March 7). Quantum Leap AI: How Gen AI optimizes portfolio structure and investment strategies. Dr. Farhad Reyazat. https://www.reyazat.com/2024/03/07/quantum-leap-ai-how-gen-ai-optimizes-portfolio-structure-and-investment-strategies/
Introduction
In the rapidly evolving landscape of the financial markets, artificial intelligence (AI) stands at the forefront of innovation, offering unprecedented opportunities for investors. As technology advances, AI has become integral to developing sophisticated investment strategies, risk management solutions, and predictive analytics tools. This integration of AI into the investment world has opened up many avenues for investors to enhance their portfolios, manage risks more effectively, and align their investment choices with their ethical values. From the high-speed world of algorithmic trading to the meticulous analysis required for sustainable investing, AI’s versatility and power reshape how we approach finance. The following summary explores the breadth of investment opportunities powered by AI, highlighting how technology is not just augmenting but fundamentally transforming the investment landscape. Whether you are an individual investor looking to diversify your portfolio with AI-driven companies and ETFs or a sophisticated trader utilizing AI for real-time market analysis and decision-making, the AI investment universe offers something for everyone. This exploration into AI and investment underscores the potential for significant returns and emphasizes the importance of technological adaptability in achieving long-term investment success.
A case study focused on an AI equity trading model called Traders’ A.I., which operated from 2019 to 2022. Here are some key findings:
- Outperformance: Traders’ A.I. outperformed its benchmark, the S&P 500, over the three years. Despite being neutral regarding long vs. short positions, its beta remained statistically zero. The AI model leveraged moments of higher skewness, achieving favorable results.
- Skewness Advantage: While the S&P 500 exhibited negative skewness (a robust left tail), Traders’ A.I. displayed positive skewness (a strong right tail). This means that the AI model had fewer days with very high returns.
- Market Timing: Interestingly, Traders’ AI avoided trading on high-return days. It seemed to anticipate high-risk premium events and refrained from taking positions. Additionally, the model performed better when going short, generating positive returns on short days while the market incurred losses.
- Bear Markets: Traders’ AI demonstrated excess performance relative to bull markets during bear markets.
The key findings from the case study on the AI equity trading model, Traders’ A.I., provide insightful observations on how artificial intelligence can create investment strategies that outperform traditional market benchmarks like the S&P 500. Here’s a breakdown of the understanding from the mentioned findings:
1. Outperformance & Neutral Beta: The AI model outperformed the S&P 500 over three years, which is a significant achievement. The neutrality regarding long vs. short positions and a beta statistically remaining at zero suggests that the AI’s performance was not reliant on market movements. Instead, it capitalized on specific opportunities, using moments of higher skewness to achieve favorable outcomes. This indicates a sophisticated risk management approach that allowed the model to gain without exposure to general market risks.
2. Skewness Advantage: in financial terms, Skewness refers to the asymmetry or bias in the distribution of returns. The S&P 500’s negative skewness (strong left tail) suggests that it tended to sudden, significant drops. Conversely, Traders’ A.I.’s positive skewness (strong right tail) indicates it had fewer but significantly high return days. This trait is advantageous because it suggests that the model managed to capture exceptional gains while avoiding the pitfalls of severe losses, leading to a more stable and upbeat performance.
3. Market Timing and Anticipation of High-risk Premium Events: The model’s ability to avoid trading on high-return days might seem counterintuitive but suggests a sophisticated strategy to prevent high volatility or overpriced assets that come with high-risk premiums. Its success in short positions during market losses indicates an effective anticipation of market downturns and the capability to profit from them, which is a valuable strategy, especially in volatile markets.
4. Performance in Bear Markets: The AI’s superior performance during bear markets compared to bull markets is particularly noteworthy. This suggests that the model was exceptionally good at identifying overvalued stocks or market conditions poised for a downturn, allowing it to take profitable short positions or avoid losses more effectively than during bull markets. This capability is crucial for portfolio management, especially for investors looking for strategies to weather or capitalize on market downturns.
Overall, Traders’ AI demonstrates the potential of AI in creating advanced trading strategies that not only manage risk effectively but also exploit specific market conditions for outperformance. Its approach to leveraging skewness, market timing, and adaptability to different market phases (bear vs. bull) highlights how AI can offer nuanced and dynamic investment strategies beyond traditional human-managed funds.
AI & investment
Category | Description | Application | Methodology |
Algorithmic Trading | Algorithmic trading involves creating trading algorithms that execute buy or sell orders based on predefined rules. These algorithms can analyze market data and execute trades at high speeds. | Prevalent in stock markets, forex, and commodities, aiming to capitalize on short-term price fluctuations. | Uses technical indicators, historical data, and real-time market information for trading decisions. |
Sentiment Analysis | AI analyzes news articles, social media posts, and other textual data to gauge market sentiment, assisting investors in making informed decisions. | Used to understand public perception of specific stocks or sectors. | Processes large volumes of text data to extract sentiment signals. |
Robo-Advisors | Robo-advisors are automated platforms that provide personalized investment advice and create and manage portfolios based on an investor’s goals and risk tolerance. | Benefits retail investors with low-cost, diversified portfolios without human intervention. | Utilizes algorithms to allocate assets and rebalance portfolios. |
Portfolio Optimization | AI algorithms optimize portfolios by considering various factors, aiming to create diversified portfolios that maximize returns while minimizing risk. | Investors seek them for efficient portfolios tailored to their risk preferences. | Employs optimization techniques to balance risk and return. |
Risk Management and Fraud Detection | AI assesses risk and detects fraud by analyzing historical data, helping investors implement risk mitigation strategies. | Utilized by institutional investors and banks for risk mitigation. | Analyzes historical data and real-time transactions for risk assessment. |
Quantitative Models | AI-driven quantitative models analyze large datasets to identify investment opportunities using factors like valuation metrics and financial ratios. | Institutional investors and hedge funds employ her for systematic trading. | Processes data with machine learning algorithms to generate buy/sell signals. |
Alternative Data Analysis | Alternative data includes non-traditional information AI analyzes to gain insights into companies or industries. | Used by hedge funds and institutional investors for alpha generation. | Processes diverse datasets with machine learning models to uncover hidden patterns. |
Factor-Based Investing | Factor-based strategies use AI to identify specific risk factors and construct portfolios to outperform market indices. | Aims to outperform market indices through a focus on specific risk factors. | Analyzes historical data with AI models to select and weigh factors. |
Deep Learning for Forecasting | Deep learning models predict asset prices or economic indicators by learning complex relationships from historical data. | They are applied in forecasting stock prices, interest rates, or macroeconomic variables. | Learns from patterns using neural networks to process sequential data. |
Sustainable Investing or Ethical AI Investing | AI evaluates ESG factors in investment decisions, helping investors align their portfolios with sustainability goals. | Aligns investor portfolios with sustainability goals. | Evaluate ESG data and rank companies with AI. |
Table 1 summarizes various AI-driven investment strategies, illustrating how artificial intelligence is applied in the financial sector to enhance investment decisions, manage risks, and optimize portfolio performance. Here’s a concise summary of the key points:
1. Algorithmic Trading: This type of trading utilizes trading algorithms to execute trades based on predefined rules. It aims to capitalize on short-term market fluctuations by analyzing market data and patterns at high speeds.
2. Sentiment Analysis: This method employs AI to analyze textual data from news and social media to gauge market sentiment, helping investors understand public perceptions of stocks or sectors.
3. Robo-Advisors: Automated platforms that offer personalized investment advice and portfolio management based on the investor’s goals and risk tolerance, providing low-cost, diversified portfolios.
4. Portfolio Optimization: AI algorithms create diversified portfolios that maximize returns while minimizing risk by considering factors such as risk tolerance and asset correlations.
5. Risk Management and Fraud Detection: Analyzes historical and real-time data to assess portfolio risk, detect anomalies, and prevent fraud, aiding in risk mitigation for investors and institutions.
6. Quantitative Models: Leverages large datasets to identify investment opportunities using valuation metrics and financial ratios, mainly employed by institutional investors and hedge funds.
7. Alternative Data Analysis: This method utilizes non-traditional data sources, such as satellite imagery or social media trends, to gain insights into companies or industries, often for alpha generation.
8. Factor-Based Investing: Focuses on specific risk factors like value, momentum, or quality, using AI to construct portfolios to outperform market indices.
9. Deep Learning for Forecasting: This method applies deep learning models to predict asset prices or economic indicators by learning from complex historical data patterns.
10. Sustainable Investing or Ethical AI Investing: Uses AI to evaluate investments based on environmental, social, and governance (ESG) factors, aligning portfolios with sustainability goals.
These strategies showcase AI’s potential to transform investment practices by offering sophisticated, data-driven approaches for analyzing markets, managing portfolios, and identifying investment opportunities.
While AI offers exciting possibilities, it’s essential to understand its limitations and risks. Investors should combine AI-driven strategies with human judgment and stay informed about market dynamics. These advancements underscore the transformative potential of AI in the investment landscape, emphasizing the need for ongoing research, ethical considerations, and regulatory engagement to realize AI’s benefits while mitigating its risks fully.
Investing in AI
The following may be regarded as various investments classified under investing in AI.
investment Type | Description |
Invest in AI Companies | Investing in stocks of public companies involved in AI software development and hardware manufacturing, focusing on those leading in AI research and implementation. |
AI Exchange-Traded Funds (ETFs) | Investing in ETFs focused on AI, holding a diversified portfolio of AI-related stocks, such as BOTZ and ARKQ. |
Algorithmic Trading Systems | Exploring AI-driven trading systems that use algorithms to analyze market data, identify patterns, and execute trades efficiently. |
Sentiment Analysis Tools | Using AI-powered tools to monitor and analyze textual data for market sentiment, aiding in informed investment decisions. |
Portfolio Optimization Models | Employing AI algorithms to optimize portfolios, considering risk tolerance, expected returns and asset correlations maximizes returns and minimizes risk. |
Risk Management Solutions | Utilizing AI to assess risk by analyzing historical data and identifying potential hazards, supporting informed risk management decisions. |
Personalized Investment Advice from Robo-Advisors | Leveraging AI-driven robo-advisors for personalized investment advice and portfolio management based on individual investor goals and risk tolerance. |
Sustainable Investing with AI | Using AI to evaluate ESG factors, identify companies with strong ESG practices, and align portfolios with sustainability goals. |
Technical Analysis Tools | Applying AI-based tools for technical analysis of historical price data, patterns, and indicators to forecast future price movements. |
Quantitative Models | Analyzing large datasets with AI-driven quantitative models to identify investment opportunities using valuation metrics, financial ratios, and historical data. |
Table 2 presents various investment opportunities within artificial intelligence (AI), catering to multiple investor preferences and objectives. These opportunities range from direct equity investments in leading AI companies at the forefront of AI software development and hardware manufacturing to more diversified approaches such as AI-focused Exchange-Traded Funds (ETFs). These ETFs encapsulate a broad spectrum of AI-related stocks, offering exposure to the sector’s growth potential.
For those interested in the dynamic aspects of the market, AI-driven algorithmic trading systems provide a technologically advanced means to analyze market data, identify trading patterns, and execute trades with precision and speed. Similarly, sentiment analysis tools leverage AI to sift through vast amounts of textual data, like news and social media, to capture market sentiment, enabling informed decision-making.
Investors seeking optimal asset allocation can benefit from portfolio optimization models that utilize AI to analyze risk tolerance, expected returns, and asset correlations. These models aim to construct diversified portfolios that balance risk and return effectively. Risk management solutions powered by AI analyze historical data to identify potential hazards, aiding in crafting robust risk mitigation strategies.
The advent of AI-driven robo-advisors has democratized access to personalized investment advice, tailoring portfolios to individual goals and risk profiles with the added advantage of automatic rebalancing. Moreover, sustainable investing with AI focuses on evaluating environmental, social, and governance (ESG) factors, allowing investors to align their portfolios with their ethical and sustainability values.
Technical analysis tools and quantitative models represent the analytical side of AI investments, offering predictions on future price movements and uncovering investment opportunities through data analysis. These tools analyze historical price data, financial ratios, and other relevant metrics to inform trading decisions.
Investing in AI spans from direct investments in AI-centric companies and ETFs to leveraging AI for sophisticated trading strategies, portfolio management, risk assessment, and achieving sustainability goals. This diversity reflects the evolving landscape of financial investments driven by AI and caters to modern investors’ varying objectives and risk appetites, promising a stake in the future of technology and innovation.
Conclusion
In conclusion, the intersection of artificial intelligence and investment represents a dynamic and rapidly advancing frontier in the financial world. AI has proven a game-changer, offering tools and strategies catering to various investment philosophies and goals. The opportunities are vast and varied, from direct investments in trailblazing AI companies to using sophisticated AI algorithms for market analysis, risk management, and portfolio optimization. Integrating AI into investing enhances the ability to make informed decisions. It opens up new pathways for achieving financial growth, managing risks more effectively, and aligning investments with ethical standards through sustainable investing practices. As we continue to navigate this exciting era of technological innovation, it becomes clear that embracing AI-driven investment strategies can significantly augment an investor’s ability to navigate complex markets. Thus, for those looking to stay ahead in the investment game, leveraging the power of artificial intelligence is not just an option; it’s becoming an indispensable part of a modern investment portfolio.
Sources:
- AI pioneers in investment management. (2019, September 30). CFA Institute. https://rpc.cfainstitute.org/en/research/reports/ai-pioneers-in-investment-management
- De Laforcade, Xavier. (2023, August 9). The impact of Artificial Intelligence on portfolio management. Rothschild & Co. https://www.rothschildandco.com/en/newsroom/insights/2023/08/rothschild-martin-maurel-the-impact-of-artificial-intelligence-on-portfolio-management/
- Wadhwani, K. (2024, February 14). A brief guide to AI in portfolio Management. Blockchain Technology, Mobility, AI and IoT Development Company USA, Canada. https://www.solulab.com/ai-in-portfolio-management/
- Bowman, J. (2023, November 14). How AI can be used in investing. The Motley Fool. https://www.fool.com/investing/stock-market/market-sectors/information-technology/ai-stocks/ai-in-investing/
- AI & Sustainable Investing: Use & Potential | Morgan Stanley. (2023 July 31). Morgan Stanley. https://www.morganstanley.com/ideas/ai-sustainable-investing-use-potential
- Katzeff, P. (2023, August 2). How to invest in AI. Forbes Advisor. https://www.forbes.com/advisor/investing/how-to-invest-in-ai/
- Grossman, A. (2023, August 19). How to invest in AI. Investopedia. https://www.investopedia.com/how-to-invest-in-ai-7504987
- Von Wilpert BBusMan, C. (2023, November 27). 7 Best AI Investing Apps 2024 (50 software tools ranked). Content Mavericks. https://contentmavericks.com/best-ai-investing-app/
- Systematic Investing. (2023, June 15). How AI is Transforming Investing | BlackRock. BlackRock. https://www.blackrock.com/us/individual/insights/ai-investing
- Filipsson, F., & Filipsson, F. (2024, February 20). Understanding AI investment strategies. Redress Compliance – Just another WordPress site. https://redresscompliance.com/understanding-ai-investment-strategies/
- Blackrock. (2023, November 9). Investor’s Guide to Artificial Intelligence (AI) | BlackRock. BlackRock. https://www.blackrock.com/us/financial-professionals/insights/investor-guide-to-ai
10 replies on “Quantum Leap AI: How Gen AI Optimizes Portfolio Structure and Investment Strategies”
[…] The advent of AI has introduced sophisticated analytical capabilities, enabling the development of d… […]
[…] For a deeper understanding, consider reading the insightful article on REYAZAT.COM titled “Quantum Leap AI: How Gen AI Optimizes Portfolio Structure and Investment Strategies.” Read more here. […]
Hi there
I have just took a look on your SEO for reyazat.com for the current search visibility and saw that your website could use a boost.
We will increase your ranks organically and safely, using only state of the art AI and whitehat methods, while providing monthly reports and outstanding support.
More info:
https://www.digital-x-press.com/unbeatable-seo/
Regards
Mike Gill
Digital X SEO Experts
I really enjoy the content thanks
Great Thank you
This is amazing thanks
The Real Person!
Author Kak zarabotat dengi_omka acts as a real person and passed all tests against spambots. Anti-Spam by CleanTalk.
как быстро заработать деньги в интернете. 400 за 15 минут kak-zarabotat-dengi11.ru .
The Real Person!
Author Trey acts as a real person and passed all tests against spambots. Anti-Spam by CleanTalk.
Good
The Real Person!
Author Mostafa acts as a real person and passed all tests against spambots. Anti-Spam by CleanTalk.
Great!
The Real Person!
Author Airen acts as a real person and passed all tests against spambots. Anti-Spam by CleanTalk.
Great one recommending for reading