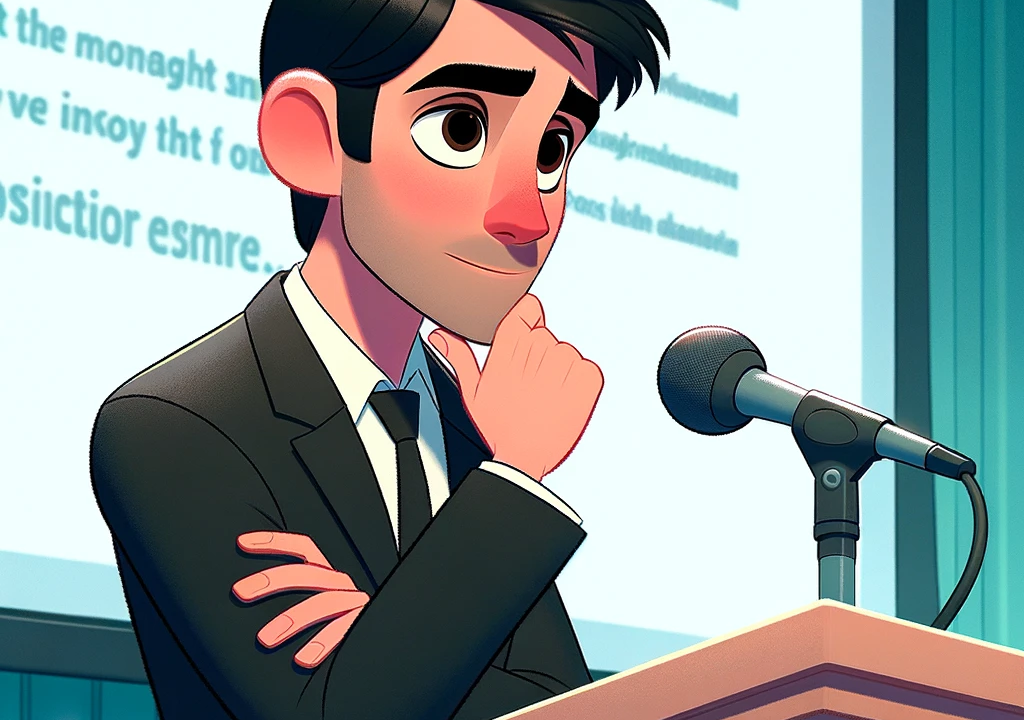
Shaping the Future of Central Banking with Artificial Intelligence (AI) and Machine Learning (ML)
Dr Farhad Reyazat; London School of Banking & Finance, London
February 2024
Citation: Reyazat, F. (2024, February 26). Shaping the Future of Central Banking with Artificial Intelligence (AI) and Machine Learning (ML). Dr. Farhad Reyazat. https://www.reyazat.com/2024/02/26/shaping-the-future-of-central-banking-with-artificial-intelligence-ai-and-machine-learning-ml/
In an era marked by unprecedented complexities in the global economy, Central Banks stand at the forefront of navigating these turbulent waters, shouldering the immense responsibility of stabilizing financial systems and fostering sustainable economic growth. As the guardians of monetary policy, these pivotal institutions increasingly leverage the transformative powers of Artificial Intelligence (AI) and Machine Learning (ML) to refine their decision-making processes and optimize policy outcomes. This convergence of finance and technology marks a significant evolution in the tools at the disposal of Central Banks and represents a paradigm shift in how economic insights are gleaned and applied. Through the sophisticated analysis of vast datasets and the predictive prowess of algorithmic models, AI and ML are reshaping the landscape of monetary policy, opening new avenues for understanding and addressing the intricate dynamics of the global economy. This introduction sets the stage for a comprehensive exploration of the innovative ways Central Banks harness AI and ML to chart a course toward more informed, efficient, and practical policy decisions, ultimately contributing to more resilient and prosperous societies.
Leveraging AI and ML for Advanced Data Analytics in Central Banking
Globally, central banks are tasked with digesting and interpreting vast volumes of diverse data, ranging from government statistics and financial surveys to market fluctuations and digital footprints left by social media interactions. In this complex data landscape, Artificial Intelligence (AI) and Machine Learning (ML) emerge as pivotal tools, equipped with sophisticated methodologies such as Natural Language Processing (NLP), Deep Learning, and Regression Trees. These technologies excel in sifting through data to unearth pertinent insights and detect underlying patterns that might elude traditional analysis.
Applying AI and ML in data analysis gives Central Banks a deeper, more immediate grasp of economic indicators, including business cycles, inflation trends, and shifts in consumer behavior. This enriched understanding bolsters the formulation and implementation of monetary policies, stabilizes financial conditions, and sharpens regulatory frameworks. For instance, the European Central Bank (ECB) has harnessed big data and ML for enhanced nowcasting and short-term predictions of real GDP, showcasing the potential of these technologies to refine economic forecasting.
Similarly, the Bank of England has integrated AI and ML into its analytical processes, yielding significant improvements in economic forecasts and financial market oversight. Their work illustrates the practical benefits of AI in extracting actionable intelligence from complex data sets, thereby informing more responsive and targeted monetary strategies.
The Reserve Bank of India (RBI) presents another compelling case, having partnered with McKinsey and Accenture to incorporate AI and ML into its regulatory supervision framework. This initiative aims to elevate the accuracy and efficiency of monitoring financial market dynamics, demonstrating a forward-thinking approach to regulatory oversight.
Such examples underscore the transformative impact of AI and ML across the central banking sector, enabling institutions to navigate the data deluge with unprecedented agility and insight. As these technologies continue to evolve, their role in shaping monetary policy, ensuring financial stability, and enhancing regulatory practices is set to expand, marking a new era of data-driven decision-making in central banking.
Enhancing Economic Forecasting Through Advanced Technologies
A pivotal task for Central Bank policymakers worldwide is accurately predicting economic trends. Traditional methods, grounded in statistical analysis, often stumble when faced with the intricate web of factors influencing economic behavior, struggling to account for the full breadth of uncertainty that characterizes economic systems. In contrast, advancements in Artificial Intelligence (AI) and Machine Learning (ML) have ushered in a new era of financial forecasting. These technologies harness the vast potential of big data, enabling the construction of models that surpass traditional approaches in both precision and reliability.
For instance, a notable study highlighted the superior performance of a machine learning model, explicitly utilizing the K-Nearest Neighbour (KNN) algorithm, over conventional time series analysis in predicting the real Gross Domestic Product (GDP) of the United States. The study revealed that this ML model provided forecasts with significantly higher accuracy, underscoring the potential of AI and ML in economic analysis. Furthermore, another investigation demonstrated the capacity of machine learning techniques to enhance demand forecasting by an impressive margin of 10 to 20 percent. Such improvements are statistically significant and carry profound implications for inventory management, supply chain optimization, and overall business strategy.
The strength of AI and ML models lies in their ability to assimilate and analyze a diverse array of predictive factors. Beyond traditional economic indicators like the yield curve and macroeconomic variables, these models can integrate data from unconventional sources, including social media sentiment and real-time financial transactions. This holistic approach allows for a more comprehensive understanding of the economic landscape, capturing the nuances and complexities that elude more straightforward statistical methods.
Moreover, adopting AI and ML in economic forecasting has practical implications across various sectors. For example, in the finance industry, these technologies can aid in identifying emerging trends, enabling more informed investment decisions. In retail, improved demand forecasting translates into optimized stock levels, reducing overstock and stockouts. The public sector, too, stands to benefit, with more accurate economic forecasts informing policy decisions and fiscal planning.
In conclusion, integrating AI and ML into economic forecasting represents a significant leap forward in our ability to predict economic trends. By leveraging big data and the sophisticated analytical capabilities of these technologies, policymakers and business leaders can make more informed decisions, steering economies and companies toward more excellent stability and growth. The evidence is clear: AI and ML are not just theoretical enhancements but practical tools that are reshaping the landscape of economic forecasting.
Leveraging AI and ML for Advanced Real-time Economic Policy Simulation by Central Banks
Integrating Artificial Intelligence (AI) and Machine Learning (ML) technologies is revolutionizing how central banks and policymakers approach economic forecasting and policy simulation. These cutting-edge tools enable real-time simulations of monetary policy impacts, offering an unprecedented capability to evaluate the potential outcomes of various policy decisions before their actual implementation. This dynamic “sandbox” environment supports refining policies to better align with intended objectives while simultaneously identifying and minimizing potential adverse effects.
One illustrative case of this technology in action involves research conducted by Deloitte, where ML algorithms were deployed to assess the ramifications of different carbon tax strategies on the United States economy. This study unveiled that implementing a revenue-neutral carbon tax could slash carbon emissions by up to 28% by 2030, alongside a notable GDP increase of 0.9%. Such findings underscore the robust analytical power of ML in dissecting and forecasting the complex interplay between environmental policy and economic performance.
Further extending the application of AI and ML beyond policy analysis, companies like DataStax are pioneering the development of real-time, AI-driven applications designed to enhance customer engagement and business strategy. These applications leverage consumer data to offer personalized recommendations, such as tailored travel packages or financial services, aligning closely with individual behaviors and preferences. This enhances the customer experience and equips businesses with the agility to adapt their offerings in tune with evolving market dynamics and consumer feedback.
Moreover, adopting AI and ML in economic policy-making extends to managing inflation rates, optimizing monetary policy, and enhancing financial stability. For instance, the Bank of England has explored machine learning to improve its forecasting models for inflation and GDP growth, showcasing the potential of these technologies to refine economic indicators and policy responses.
The potential of AI and ML in economic policy simulation and analysis is vast, ranging from environmental and fiscal policy optimization to real-time business strategy adaptation and enhanced customer service. As central banks and businesses harness these technologies, the scope for more informed, effective, and responsive policy-making and strategic planning is significantly broadened. The implications for economic stability, environmental sustainability, and business innovation are profound, marking a pivotal shift in how economic outcomes are modeled, predicted, and influenced in the digital age.
AI and ML enable the simulation of economic policy outcomes in real time, allowing policymakers to evaluate the potential impacts of different decisions before implementation. This “sandbox” approach helps fine-tune policies to ensure they are as effective as possible, minimizing unintended consequences.
Financial Market Oversight with AI and ML
In the fast-paced and intricately connected world of financial markets, staying ahead of trends, anomalies, and potential risks is paramount for central banks and regulatory authorities. These markets are complex and produce vast volumes of data every second, encompassing market prices, trading activities, news feeds, social media commentary, and much more. The challenge of monitoring this ceaseless flow of information for signs of instability, fraud, or market manipulation is monumental. However, the advent of artificial intelligence (AI) and machine learning (ML) technologies has dramatically enhanced the capabilities of these institutions to perform real-time surveillance and risk assessment.
AI and ML tools are adept at sifting through these large and complex datasets, employing sophisticated algorithms such as neural networks, deep learning, and natural language processing to discern patterns and insights imperceptible to human analysts. These technologies detect nuanced signs of market irregularities, including fraudulent transactions, financial crimes, market manipulation tactics like spoofing, and emerging cyber threats.
For example, the U.S. Securities and Exchange Commission (SEC) leverages AI and ML in its Market Abuse Unit to identify suspicious trading patterns across millions of transactions, which may indicate manipulative behaviors or insider trading. Similarly, the European Central Bank (ECB) utilizes these technologies to monitor payment systems for signs of cyber-attacks, ensuring the security and integrity of financial transactions within the Eurozone.
Moreover, AI and ML enhance economic stability and resilience by providing central banks with the tools to predict and mitigate potential financial crises. By analyzing market sentiment indicators extracted from news articles and social media, central banks can gauge the market’s mood and anticipate volatile movements before they materialize. For instance, the Bank of England has developed machine learning models to predict bank stress better and assess the financial system’s overall health, allowing for more informed policy decisions.
Applying AI and ML in financial market monitoring has also led to regulatory compliance and enforcement innovations. Regulatory technology (RegTech) solutions powered by AI assist financial institutions in meeting compliance requirements more efficiently and effectively, reducing the risk of costly violations.
In summary, AI and ML have become indispensable tools for central banks and financial regulators, enabling them to navigate the complexities of modern financial markets with unprecedented precision and agility. By harnessing these technologies, institutions can protect the financial system from various risks, from market manipulation to cyber threats, safeguarding economic stability and fostering a resilient financial ecosystem.
Transforming Banking with AI and ML: Towards Personalization and Efficiency
The advent of Artificial Intelligence (AI) and Machine Learning (ML) has catalyzed a transformative shift in the banking sector, far beyond enhancing policy decision-making. These technologies are at the forefront of revolutionizing customer service, enabling financial institutions to offer highly personalized banking experiences unimaginable a few decades ago. Through the sophisticated analysis of vast amounts of customer data, banks are now capable of tailoring services to individual needs with unprecedented precision.
AI and ML excel in dissecting and understanding patterns within customer data, from transaction histories to online banking behaviors. This deep analysis allows banks to offer personalized product recommendations, such as loans with interest rates that reflect the customer’s financial history and risk profile or investment opportunities aligned with their spending habits and savings goals. For example, by leveraging AI, JPMorgan Chase has improved customer interaction by providing personalized financial advice and increasing customer satisfaction and engagement.
Moreover, the role of AI in fraud detection exemplifies the dual benefits of enhanced security and customer trust. By analyzing transaction patterns in real-time, AI systems can identify anomalies that may indicate fraudulent activity, thereby protecting customers’ assets more effectively. Citibank, for instance, utilizes ML algorithms to detect unusual patterns across millions of transactions, significantly reducing the incidence of fraudulent activities and enhancing customer trust.
Integrating AI and ML in banking extends to automating customer service interactions through advanced chatbots and virtual assistants. These AI-driven systems offer customers 24/7 access to banking services, from checking account balances to initiating transactions, without human intervention. Bank of America’s Erica, a virtual financial assistant, has successfully handled millions of customer queries, showcasing the scalability and efficiency of AI in customer service.
The impact of AI and ML on banking is also evident in regulatory compliance. By automating the monitoring and reporting of transactions, AI significantly reduces compliance burden, ensuring banks adhere to financial regulations more efficiently. This minimizes the risk of costly regulatory fines and allows banks to allocate more resources toward innovation and customer service.
In conclusion, incorporating AI and ML into the banking sector is not just a futuristic concept but a present reality reshaping the financial services landscape. From offering personalized banking solutions and enhancing fraud detection to improving regulatory compliance and customer service, AI and ML are pivotal in driving the industry toward greater efficiency, security, and customer satisfaction. As banks continue to harness these technologies, the future of banking promises even more innovative and customer-centric services, solidifying the role of AI and ML as critical enablers of the financial industry’s evolution.
Complex Landscape of AI and ML in Central Banking: Challenges and Pathways
Integrating Artificial Intelligence (AI) and Machine Learning (ML) into Central Bank operations heralds a transformative era with the promise of unprecedented efficiencies and capabilities. However, this journey is not without its hurdles. The challenges are multifaceted, encompassing ethical, social, technical, and regulatory dimensions, requiring meticulous attention and strategic management.
- Ethical and Social Implications
One of the primary concerns revolves around ethical and social considerations. Protecting data privacy is paramount, as AI and ML systems process vast amounts of personal and financial information. The European Union’s General Data Protection Regulation (GDPR) provides a robust framework, emphasizing the importance of safeguarding personal data and ensuring transparency in AI-driven decisions. Furthermore, the accountability and explainability of algorithms are critical, as the decisions of these systems can significantly impact financial markets and individual lives. Initiatives like DARPA’s Explainable AI (XAI) program aim to create more interpretable AI models without sacrificing performance.
The fairness and inclusiveness of AI and ML outcomes also demand scrutiny. Studies have shown that AI systems can inadvertently perpetuate biases in their training data, leading to unfair outcomes. For instance, a research investigation highlighted that specific credit-scoring AI models were less favorable to minority groups, underscoring the need for bias mitigation strategies in AI development.
- Technical and Operational Challenges
On the technical front, ensuring the quality and reliability of data is a prerequisite for effective AI and ML applications. Inaccuracies in data can lead to flawed insights, making robust data governance practices essential. The Bank of England, for example, has initiated projects to improve data quality and accessibility, facilitating more accurate AI-powered analyses.
The resilience of models under varying conditions is another technical challenge. The COVID-19 pandemic highlighted the need for models adapting to rapidly changing economic landscapes. Central Banks, such as the Federal Reserve, are exploring adaptive learning algorithms that can update their predictions based on new data, enhancing their robustness.
Aligning AI and ML objectives with Central Bank mandates requires a careful balance between innovation and the core values of stability and trust. For instance, the Swiss National Bank has been actively researching the potential of AI in detecting fraudulent activities while ensuring that these technologies align with its broader goals of financial stability.
- Regulatory Frameworks
The regulatory landscape for AI and ML in banking is still evolving. A clear and consistent framework is essential to foster innovation while mitigating risks. The Financial Stability Board (FSB) has been working on guidelines that address the use of AI and ML in financial services, emphasizing the importance of transparency, fairness, and ethical use.
Central Banks must navigate these challenges in collaboration with a broad spectrum of stakeholders, including financial institutions, technology providers, regulators, and the public. Engaging in open dialogues, sharing best practices, and developing common standards can facilitate the responsible and effective adoption of AI and ML. For example, the International Monetary Fund (IMF) and the World Bank have hosted forums to discuss the implications of AI in financial stability, encouraging global cooperation.
In conclusion, while AI and ML present substantial opportunities for enhancing Central Bank operations, the path forward requires a nuanced approach to address ethical, technical, and regulatory challenges. By fostering collaboration and adopting a balanced and principled approach, Central Banks and stakeholders can harness the full potential of AI and ML for the common good, ensuring a future where technology serves as a cornerstone of financial stability and prosperity.
Conclusion
In conclusion, the integration of Artificial Intelligence (AI) and Machine Learning (ML) by Central Banks signifies a monumental shift in the approach to monetary policy and economic stewardship. Through the strategic deployment of these advanced technologies, Central Banks are not only enhancing their analytical capabilities but are also setting new standards for predictive accuracy and policy effectiveness. This evolution underscores a proactive commitment to embracing the potential of digital transformation to address contemporary economic challenges. As AI and ML continue to evolve, their role in shaping monetary policies is expected to deepen, promising a future where economic stability and growth are increasingly informed by data-driven insights and sophisticated algorithms. Thus, the journey of Central Banks into the realms of AI and ML not only marks a pivotal advancement in financial governance but also serves as a beacon for innovation, efficiency, and resilience in the face of global economic uncertainties.
References:
- Frontiers in Artificial Intelligence. (2021). GDP Forecasting: Machine Learning, Linear or Autoregression? Retrieved from https://www.frontiersin.org/articles/10.3389/frai.2021.757864/full
- Anaplan. (n.d.). Demystifying AI and ML-based Forecasting. Retrieved from https://www.anaplan.com/blog/demystifying-ai-and-ml-based-forecasting/
- Wasserbacher, H., & Spindler, M. (2021). Recent developments and pitfalls include machine learning for financial forecasting, planning, and analysis. Digital Finance, 4(1), 63–88. https://doi.org/10.1007/s42521-021-00046-2
- Mariani, J. (2023, June 7). AI for smarter legislation. Deloitte Insights. https://www2.deloitte.com/us/en/insights/industry/public-sector/artificial-intelligence-can-benefit-the-legislative-process.html
- Real-Time AI | DataStax. (n.d.). DataStax. https://www.datastax.com/products/real-time-ai
- FinChat.io. (2023, August 2). The 14 best AI tools for finance – FinChat.io. https://finchat.io/blog/best-ai-tools-for-finance/
- Firmai. (n.d.). GitHub – format/financial-machine-learning: A curated list of practical financial machine-learning tools and applications. GitHub. https://github.com/firmai/financial-machine-learning
- Kreger, A. (2023, March 20). The future of AI in banking. Forbes. https://www.forbes.com/sites/forbesbusinesscouncil/2023/03/20/the-future-of-ai-in-banking/?sh=22d910355ed5
- Admin. (2023, November 22). AI and Machine Learning for Core Banking Excellence – Stefanini. Stefanini. https://stefanini.com/en/insights/articles/ai-and-machine-learning-for-core-banking-excellence
- Biswas, S., Carson, B., Chung, V., Singh, S., & Thomas, R. (2020, September 19). AI-bank of the future: Can banks meet the AI challenge? McKinsey & Company. https://www.mckinsey.com/industries/financial-services/our-insights/ai-bank-of-the-future-can-banks-meet-the-ai-challenge
- Hirschbühl, D. (2021, August 4). Using machine learning and big data to analyse the business cycle. European Central Bank. https://www.ecb.europa.eu/pub/economic-bulletin/articles/2021/html/ecb.ebart202105_02~c429c01d24.en.html
- Newsletter on artificial intelligence and machine learning. (2022, March 16). https://www.bis.org/publ/bcbs_nl27.htm
- Machine learning at central banks. (2017, September 1). Bank of England. https://www.bankofengland.co.uk/working-paper/2017/machine-learning-at-central-banks
- Biswas, S., Carson, B., Chung, V., Singh, S., & Thomas, R. (2020b, September 19). AI-bank of the future: Can banks meet the AI challenge? McKinsey & Company. https://www.mckinsey.com/industries/financial-services/our-insights/ai-bank-of-the-future-can-banks-meet-the-ai-challenge
- Sekhar, V., & Hobbs, B. (2023, October 19). What banking directors should ask about AI and machine learning risks. https://www.ey.com/en_us/board-matters/banking-risks-from-ai-and-machine-learning
No Comments