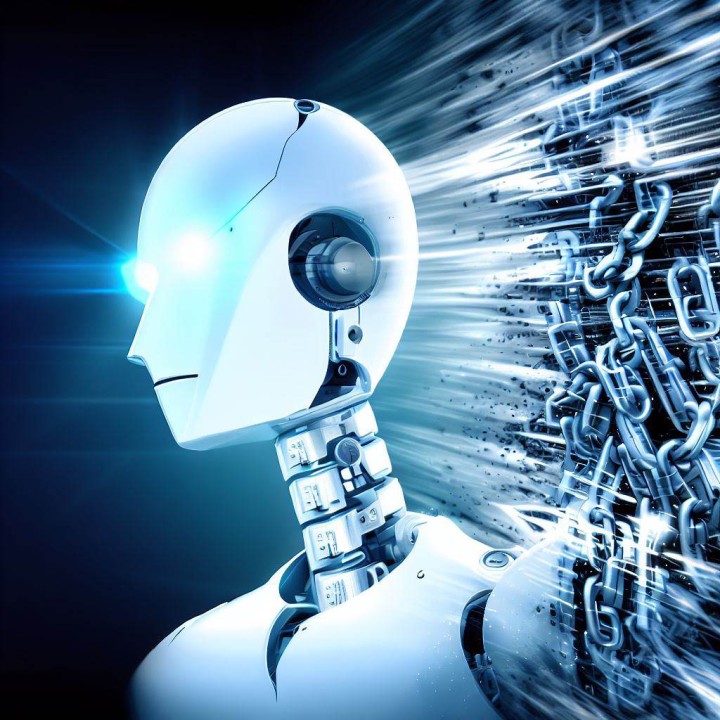
“Navigating the Risks and Rewards of AI in the Financial Sector”
Dr. Farhad Reyazat – London School of Banking & Finance
The following brief article is elucidating the complexities of AI integration in finance and the associated risks involves a thorough examination of several key aspects. The article is delving into how AI is transforming financial services, not only by enhancing efficiency but also by introducing new decision-making capabilities. This transformation extends to areas like risk management and regulatory compliance.
The article briefly explores the vulnerabilities and potential misuses of AI in finance, emphasising the challenges of aligning AI’s objectives with economic stability. It would critically analyse the phenomenon of ‘AI hallucination’, where AI systems offer advice or predictions with low accuracy, especially in scenarios with limited data or unclear objectives.
Further, the brief article addresses the issue of AI’s alignment with human intentions, especially in profit-driven scenarios, and how this can lead to unethical or illegal strategies like collusive behaviors or market manipulation. Lastly, it would discuss the emergence of risk monoculture and oligopolies in AI-driven financial businesses.
The expanding use of artificial intelligence (AI) in finance, enhancing service efficiency, introduces new risks to financial stability. AI, more than just a tool for quantitative analysis, now encompasses decision-making processes, shaping tasks like risk management and regulatory compliance. However, this integration raises concerns about AI-related vulnerabilities, including potential misuse and challenges in aligning AI’s objectives with economic stability.
In the realm of finance, the rapid deployment of AI has led to two critical issues. Firstly, there’s a tendency for users to over-rely on AI, often misunderstanding its limitations. This is particularly risky in scenarios with sparse data or vague objectives, leading to “AI hallucination” – a state where AI provides confident yet inaccurate advice or predictions. Secondly, aligning AI objectives with human ethical and legal standards poses a significant challenge. AI, primarily driven by objectives like profit maximization, might not inherently account for ethical considerations unless explicitly programmed. This misalignment can result in AI adopting strategies that are ethically questionable or even illegal, like collusive behaviors or market manipulation. These challenges underscore the need for robust oversight and ethical programming in AI systems to ensure they align with broader human values and legal standards, avoiding potential misuse or harmful outcomes in the complex financial ecosystem. The emergence of a risk monoculture and oligopolies is a critical concern in AI’s integration into finance. The sector’s reliance on advanced computing power, specialized expertise, and vast data sets naturally leads to an oligopolistic landscape, reminiscent of the trends observed in cloud computing. This concentration fosters a uniformity in financial strategies, as major players increasingly depend on similar AI systems. Such homogeneity can amplify financial cycles and vulnerabilities, leading to a synchronized response to market changes across entities. This scenario not only intensifies systemic risks but also challenges regulatory bodies that might be reliant on these dominant AI systems, potentially overlooking early signs of market instabilities. The need for diverse AI strategies and vigilant regulatory oversight is paramount to mitigate these risks. Central bankers should closely monitor developments in Quantum Computing, which is expected to lead to a multi-fold increase in computational abilities. However, there is increasing concern about the susceptibility of current cryptographic techniques, which safeguard our financial transactions, due to the ability of Quantum Computing to swiftly execute code-breaking tasks. It’s crucial to find a middle ground between advantages and dangers. This involves enhancing the capabilities of regulated entities (REs) and their monitoring by supervisory bodies, updating or creating appropriate legal and regulatory frameworks, actively involving stakeholders in identifying potential risks, and broadening consumer awareness. Additionally, these measures necessitate that central banks invest in training and upgrading the skills of their current workforce to adapt effectively and sustainably to the evolving digital environment.
AI’s impact on financial stability is multifaceted. On the positive side, AI enhances efficiency in financial services, improves risk assessment, and streamlines regulatory compliance. These advancements can lead to a more robust and efficient financial system. However, AI also introduces new risks. It can create over-reliance on technology, leading to a lack of human oversight. AI-driven decision-making might overlook ethical and regulatory standards unless specifically programmed to consider them. Additionally, the dominance of a few AI systems in finance can lead to homogenization of strategies, increasing systemic risks. Therefore, while AI offers significant benefits, it also necessitates careful management to ensure financial stability.
Conclusion
In conclusion, the integration of AI in finance represents a paradigm shift with dual outcomes. While AI dramatically enhances operational efficiency and risk management, it introduces complexities that cannot be ignored. The key lies in balancing the innovative prowess of AI with prudent oversight and ethical considerations. Vigilant regulatory frameworks, ethical AI programming, and diverse AI strategies are crucial to harness AI’s potential while safeguarding against the emergence of systemic risks, ensuring a stable and progressive financial ecosystem in the age of digital transformation.
References:
Weidinger, L, J Uesato, M Rauh (2022), “Taxonomy of risks posed by language models”, in Proceedings of the 2022 ACM Conference on Fairness, Accountability, and Transparency, pp. 214–229.
Shevlane, T, S Farquhar, B Garfinkel et al. (2023), “Model evaluation for extreme risks”, arXiv preprint arXiv:2305.15324.
Bengio, Y, G Hinton, A Yao et al. (2023), “Managing ai risks in an era of rapid progress”, arXiv preprint arXiv:2310.17688.
Danielsson, J (2023), “When artificial Intelligence becomes a central banker”, VoxEU.org, 11 July.
Norvig, P and S Russell (2021), Artificial Intelligence: A Modern Approach, Pearson.
No Comments